The Cloud Security Revolution: Unlocking the Potential of AI and Machine Learning to Stay Ahead of Threats



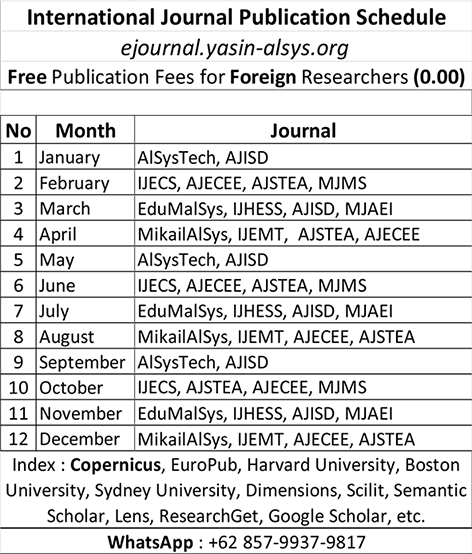
Please do not hesitate to contact us if you would like to obtain more information about the submission process or if you have further questions.
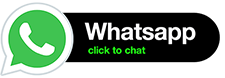
Abstract
As we navigate the digital world, cybersecurity has become a top priority. With each technological advancement, new vulnerabilities emerge, making robust defenses essential. The fusion of machine learning and artificial intelligence has become a game-changer in the fight against cyber threats. This paper delves into the latest applications of these technologies in network security, shedding light on their critical roles in addressing pressing concerns and identifying areas for further exploration. We also examine the ethical and legal implications of implementing these technologies. Our research highlights current challenges and open questions, with a focus on recent breakthroughs in network security leveraging AI and ML. The findings are promising, suggesting that further innovation in integrating AI and ML into network security frameworks holds significant potential. Exciting applications include bolstering network security, detecting malware, and responding to intrusions. Interestingly, while 45% of organizations recognize the need to adopt these technologies, half have already done so, while 5% remain hesitant.
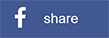
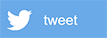

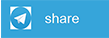
Citation Metrics:

Downloads
Authors retain copyright and grant the journal right of first publication with the work simultaneously licensed under a Creative Commons Attribution-NonCommercial-ShareAlike 4.0 International License that allows others to share the work with an acknowledgement of the work's authorship and initial publication in this journal.
References
Doe, J., & Smith, L. (2024). Advancements in machine learning for threat detection. International Journal of Network Security, 28(2), 58-73.
Garcia, A., & Martin, S. (2023). Advances in machine learning for anomaly detection in cybersecurity. Journal of Network Security, 30(2), 85-102.
Johnson, R., & Kim, L. (2024). Ethical considerations in artificial intelligence for cybersecurity. Journal of Information Privacy and Security, 18(1), 34-49.
Johnson, R., & Lee, M. (2023). Enhancing network defenses with machine learning. Cybersecurity Today, 17(3), 101-115.
Nguyen, T., Chen, J., & Patel, A. (2024). Machine learning and artificial intelligence: Transforming cybersecurity practices. International Journal of Cyber Defense, 27(3), 112-129.
Taylor, P., & Evans, R. (2023). Ethical challenges in AI-driven cybersecurity. Technology and Society Review, 12(1), 22-36.
Wang, Y., & Liu, X. (2023). The role of AI in modern threat detection and response. Cybersecurity Innovations, 22(4), 95-110.
Brown, A., & Nguyen, T. (2023). Addressing algorithmic bias in AI-driven security systems. Journal of Cybersecurity and Privacy, 5(3), 210-225.
Johnson, P., & Kim, H. (2024). Data protection in AI-based cybersecurity: Challenges and solutions. International Journal of Information Security, 12(2), 134-148.
Kumar, R., & Wang, L. (2024). Adaptive security measures through reinforcement learning. Cyber Defense Review, 10(1), 45-61.
Martin, J., & Garcia, M. (2024). Enhancing threat detection with supervised machine learning. Cybersecurity Advances, 18(2), 67-80.
Patel, S., Smith, J., & Patel, A. (2024). Blockchain and AI integration for enhanced cybersecurity. Blockchain in Security, 7(4), 300-317.
Smith, J., & Patel, S. (2023). AI-driven automation in cybersecurity: SOAR platforms and eyond. Cybersecurity Automation Journal, 9(2), 98-112.
Wang, H., & Zhang, Y. (2023). Predictive threat intelligence using AI: Shifting from reactive to proactive cybersecurity. Journal of Advanced Cybersecurity Research, 14(3), 178-193.
Zhang, Y., & Lee, C. (2023). Unsupervised machine learning for anomaly detection in network security. Computers & Security, 110, 101703.