Data-Driven Education: Data Processing as a Key to Improving the Quality of Mathematics Education




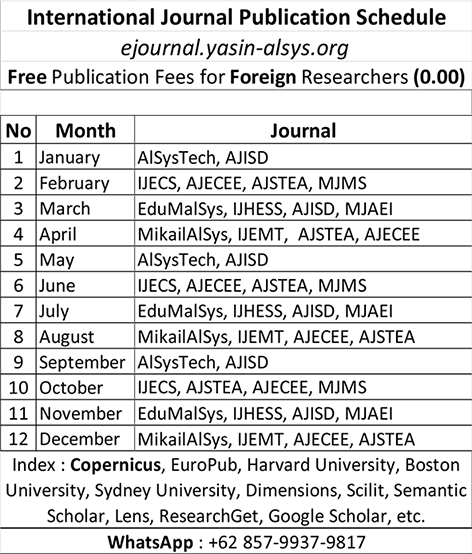
Please do not hesitate to contact us if you would like to obtain more information about the submission process or if you have further questions.
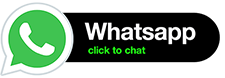
Abstract
Education is a major pillar in the development of a nation, and the quality of education becomes a key factor in determining the progress of a society. In this digital era, there is a rapid development in the use of information technology, and this has a significant impact on the education sector. The purpose of this study is for data-driven education: data processing as the key to improving the quality of mathematics education. This research uses a mixed method that combines qualitative and quantitative methods. This approach was chosen to address the complexity of data management and the comprehensiveness of this topic. The research begins with an in-depth literature analysis to understand the potential of Data-Driven Education. By using data as a guide, educators can make smarter decisions, detail instructional strategies, and create an educational environment that allows each student to reach his or her full potential. This is done by combining the results of various data of educator learners who can make informational decisions to improve the quality of mathematics learning. The application of data management in the context of mathematics education can provide concrete solutions to overcome challenges and improve the quality of learning. The right use of data provides the basis for smarter decisions and more adaptive learning, creating a learning environment that fits the needs of each student. By integrating Data-Driven Education effectively, we can move towards a more inclusive, personalized and successful mathematics education. The use of data contributes significantly in improving the quality and inclusivity of mathematics education. The integration of data management in mathematics education is a critical step towards a more adaptive, inclusive, and effective education
Downloads
Authors retain copyright and grant the journal right of first publication with the work simultaneously licensed under a Creative Commons Attribution-NonCommercial-ShareAlike 4.0 International License that allows others to share the work with an acknowledgement of the work's authorship and initial publication in this journal.
References
Akhtar, Pervaiz, Jędrzej George Frynas, Kamel Mellahi, and Subhan Ullah. (2019). “Big Data-Savvy Teams’ Skills, Big Data-Driven Actions and Business Performance.” British Journal of Management 30(2):252–71. doi: 10.1111/1467-8551.12333.
Gao, Peng, Jingyi Li, and Shuai Liu. (2021). “An Introduction to Key Technology in Artificial Intelligence and Big Data Driven E-Learning and e-Education.” Mobile Networks and Applications 26(5):2123–26. doi: 10.1007/s11036-021-01777-7.
Hamoud, Alaa Khalaf, Marwah Kamil Hussein, Zahraa Alhilfi, and Rabab Hassan Sabr. (2021). “Implementing Data-Driven Decision Support System Based on Independent Educational Data Mart.” International Journal of Electrical and Computer Engineering 11(6):5301–14. doi: 10.11591/ijece.v11i6.pp5301-5314.
Hora, Matthew T., Jana Bouwma-Gearhart, and Hyoung Joon Park. (2017). “Data Driven Decision-Making in the Era of Accountability: Fostering Faculty Data Cultures for Learning.” Review of Higher Education 40(3):391–426. doi: 10.1353/rhe.2017.0013.
Levin, James A., and Amanda Datnow. (2012). “The Principal Role in Data Driven Decision Making: Using Case Study Data to Develop Multi?Mediator Models of Educational Reform.” School Effectiveness and School Improvement 23(2):179–201.
Lv, Zongming. (2021). “The Design of Mathematics Teaching Optimization Scheme Based on Data-Driven Decision-Making Technology.” Scientific Programming 2021. doi: 10.1155/2021/5377784.
Mandinach, Ellen B. (2012). “A Perfect Time for Data Use: Using Data-Driven Decision Making to Inform Practice.” Educational Psychologist 47(2):71–85. doi: 10.1080/00461520.2012.667064.
Mendez, Gonzalo, Xavier Ochoa, Katherine Chiluiza, and Bram De Wever. (2014). “Curricular Design Analysis: A Data-Driven Perspective.” Journal of Learning Analytics 1(3):84–119. doi: 10.18608/jla.2014.13.6.
Ronka, David, Robb Geier, and Malgorzata Marciniak. (2010). “A Practical Framework for Building a Data-Driven District or School : How a Focus on Data Quality , Capacity , and Culture Supports Data-Driven Action To Improve Student Outcomes.” PCG Education White Paper (617):4–10.
Sarker, Iqbal H. (2021). “Data Science and Analytics: An Overview from Data-Driven Smart Computing, Decision-Making and Applications Perspective.” SN Computer Science 2(5):1–22. doi: 10.1007/s42979-021-00765-8.
Sugiono. (2012). Metode Penelitian Kuantitatif, Kualitatif, Dan R&D. Bandung: Alfabeta CV.
Sun, Qingqiang, and Zhiqiang Ge. (2021). “A Survey on Deep Learning for Data-Driven Soft Sensors.” IEEE Transactions on Industrial Informatics 17(9):5853–66. doi: 10.1109/TII.2021.3053128.
Teng, Sin Yong, Michal Touš, Wei Dong Leong, Bing Shen How, Hon Loong Lam, and Vítězslav Máša. (2021). “Recent Advances on Industrial Data-Driven Energy Savings: Digital Twins and Infrastructures.” Renewable and Sustainable Energy Reviews 135(August 2020). doi: 10.1016/j.rser.2020.110208.
Yazici, Mahmut, Shadi Basurra, and Mohamed Gaber. (2018). “A DATA-DRIVEN ARCHITECTURE FOR PERSONALIZED QOE MANAGEMENT IN 5G WIRELESS NETWORKS.” Big Data and Cognitive Computing 2(4):1–11.
Yin, Feng, Zhidi Lin, Qinglei Kong, Yue Xu, Deshi Li, Sergios Theodoridis, and Shuguang Robert Cui. (2020). “FedLoc: Federated Learning Framework for Data-Driven Cooperative Localization and Location Data Processing.” IEEE Open Journal of Signal Processing 1(May):187–215. doi: 10.1109/OJSP.2020.3036276.
Zhang, Junping, Fei Yue Wang, Kunfeng Wang, Wei Hua Lin, Xin Xu, and Cheng Chen. (2011). “Data-Driven Intelligent Transportation Systems: A Survey.” IEEE Transactions on Intelligent Transportation Systems 12(4):1624–39. doi: 10.1109/TITS.2011.2158001.