Fuzzy Arithmetic–Based Algorithm for Identifying Medical Conditions for Better Treatment




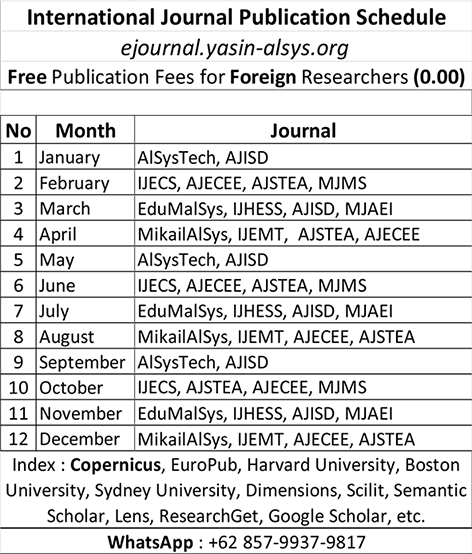
Please do not hesitate to contact us if you would like to obtain more information about the submission process or if you have further questions.
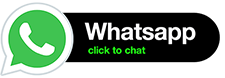
Abstract
Making the right medical decision is challenging work because, in our daily life, decision-making problems may have the components of membership and non-membership degrees with the possibility of hesitation. Since soft theory offers a theoretical framework for dealing with ambiguous, fuzzy, and ill-defined objects, it is a key development in the field of computer programming as well as other scientific disciplines. Intuitionistic fuzzy soft sets provide an effective tool for solving multiple attribute decision-making with intuitionistic fuzzy information. The most essential issue is how to derive the ranking of alternatives from the information quantified in terms of intuitional fuzzy values. This theory also has the potential to be used to solve such real-world problems. In this work, we explore how Sanchez's medical theory could be used in medical diagnosis and provide a fuzzy arithmetic-based algorithm for identifying medical conditions to address this.
Citation Metrics:

Downloads
Authors retain copyright and grant the journal right of first publication with the work simultaneously licensed under a Creative Commons Attribution-NonCommercial-ShareAlike 4.0 International License that allows others to share the work with an acknowledgement of the work's authorship and initial publication in this journal.
References
Zadeh, L. A. (1965). Fuzzy sets. Information and control, 8(3), 338-353.
Hndoosh, R., Saroa, M., & Kumar, S. (2012). Fuzzy and adaptive neuro-fuzzy inference system of washing machine. European Journal of Scientific Research, 86(3), 443-459.
Kumar, S., & Jain, H. (2012). A fuzzy logic-based model for life insurance underwriting when insurer is diabetic. European Journal of Applied Sciences, 4(5), 196-202.
Allahverdi, N., & Ertosun, S. (2018). Application of fuzzy logic for risk determination of type-2 diabetes disease. In 6th International Conference on Control and Optimization with Industrial Applications. Baku, Azerbaijan.
Paudel, G. P., Pahari, N. P., & Kumar, S. (2022). Application of fuzzy logic through Bellmen-Zadeh maximin method. Journal of Nepal Mathematical Society, 5(1), 41-47.
Atanassov, K. (1988). Review and new results on intuitionistic fuzzy sets. preprint Im-MFAIS-1-88, Sofia, 5(1).
Ejegwa, P. A., & Onasanya, B. O. (2019). Improved intuitionistic fuzzy composite relation and its application to medical diagnostic process. Note IFS, 25(1), 43-58.
Ejegwa, P. A., & Onyeke, I. C. (2020). Medical diagnostic analysis on some selected patients based on modified Thao et al.’s correlation coefficient of intuitionistic fuzzy sets via an algorithmic approach. Journal of fuzzy extension and applications, 1(2), 130-141.
Aggarwal, H., Arora, H. D., & Kumar, V. (2019). A decision-making problem as an application of intuitionistic fuzzy set. Int. J. Eng. Adv. Technol, 9, 5259-5261.
Adamu, I. M. (2021). Application of intuitionistic fuzzy sets to environmental management. Notes on Intuitionistic Fuzzy Sets, 27(3), 40-50.
Molodtsov, D. (1999). Soft set theory—first results. Computers & mathematics with applications, 37(4-5), 19-31.
Yang, X., Yu, D., Yang, J., & Wu, C. (2007). Generalization of soft set theory: from crisp to fuzzy case. In Fuzzy Information and Engineering, 345-354.
Deli, I., & Çağman, N. (2015). Intuitionistic fuzzy parameterized soft set theory and its decision making. Applied Soft Computing, 28, 109-113.
Hooda, D. S., Kumari, R., & Sharma, D. K. (2018). Intuitionistic Fuzzy Soft Set Theory and Its Application in Medical Diagnosis. International Journal of Statistics in Medical Research, 7(3), 70-76.
Maji, P. K., Biswas, R., & Roy, A. R. (2001). Intuitionistic fuzzy soft sets. Journal of fuzzy mathematics, 9(3), 677-692.
Maji, P. K., Roy, A. R., & Biswas, R. (2002). An application of soft sets in a decision making problem. Computers & Mathematics with Applications, 44(8-9), 1077-1083.
Maji, P. K., Biswas, R., & Roy, A. R. (2003). Soft set theory. Computers & Mathematics with Applications, 45(4-5), 555-562.
Neog, T. J., & Sut, D. K. (2011). Theory of fuzzy soft sets from a new perspective. International Journal of Latest Trends in Computing, 2(3), 439-450.
Feng, F., Xu, Z., Fujita, H., & Liang, M. (2020). Enhancing PROMETHEE method with intuitionistic fuzzy soft sets. International Journal of Intelligent Systems, 35(7), 1071-1104.
Hu, J., Pan, L., Yang, Y., & Chen, H. (2019). A group medical diagnosis model based on intuitionistic fuzzy soft sets. Applied soft computing, 77, 453-466.
Sanchez, E. (1976). Resolution of composite fuzzy relation equations. Information and control, 30(1), 38-48.