Ensemble Machine Learning Algorithm for Diabetes Prediction in Maiduguri, Borno State




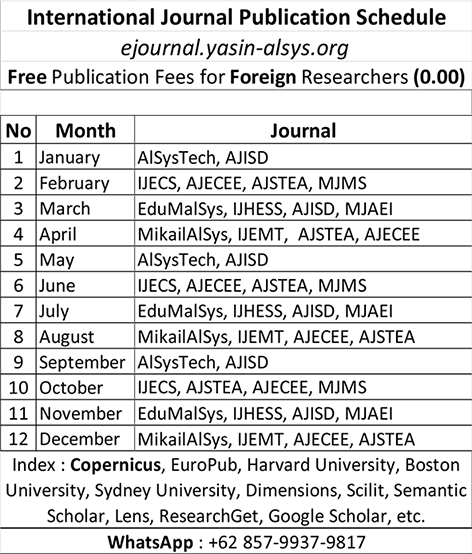
Please do not hesitate to contact us if you would like to obtain more information about the submission process or if you have further questions.
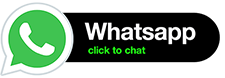
Abstract
Diabetes mellitus (DM) is a metabolic disease characterised by high levels of glucose in the blood, known as hyperglycemia, that can result in multiple problems within the body. The World Health Organisation (WHO) data for 2021 reveals a substantial increase in the prevalence of diabetes mellitus (DM), with the number of cases rising from 108 million in 1980 to 422 million in 2014. Between 2000 and 2019, there was a 3% increase in mortality rates associated with diabetes, categorised by age. In 2019, DM caused the deaths of more than 2 million people. These concerning figures clearly necessitate an immediate response. An alarming incidence of diabetes among the population of Maiduguri and Borno State inspired this investigation. This research proposed stacking ensemble learning approach to predict the rate of occurrence of diabetes cases in Maiduguri. The paper used different types of regression models to predict the occurrences of diabetes cases in Maiduguri over time. These models included adaptive boosting regression (Adaboost), gradient boosting regression (GBOOST), random forest regression (RFR), ordinary least square regression (OLS), least absolute shrinkage selection operator regression (LASSO), and ridge regression (RIDGE). The performance indicators studied in this work are root mean square (RMSE), mean absolute error (MAE), and mean square error (MSE). These metrics were used to assess the effectiveness of both the machine learning and proposed Stacking Ensemble Learning (SEL) approaches. Performance metrics considered in this study are root mean square (RMSE), mean absolute error (MAE), and mean square error (MSE), which were used to evaluate the performance of the machine learning and the proposed Stacking Ensemble Learning (SEL) technique. Experimental results revealed that SEL is a better predictor compared to other machine learning approaches considered in this work with an RMSE of 0.0493; a MSE of 0.0024; and a MAE of 0.0349. It is hoped that this research will help government officials understand the threat of diabetes and take the necessary mitigation actions.
Citation Metrics:

Downloads
Authors retain copyright and grant the journal right of first publication with the work simultaneously licensed under a Creative Commons Attribution-NonCommercial-ShareAlike 4.0 International License that allows others to share the work with an acknowledgement of the work's authorship and initial publication in this journal.
References
Abdulhadi, N., & Al-Mousa, A. (2021, July). Diabetes detection using machine learning classification methods. In 2021 International Conference on Information Technology (ICIT) (pp. 350-354). IEEE.
Adeleye, J. O. (2021). The hazardous terrain of diabetes mellitus in Nigeria: the time for action is now. Research Journal of Health Sciences, 9(1), 69-76.
Alasaady, M. T., Aris, T. N. M., Sharef, N. M., & Hamdan, H. (2022). A proposed approach for diabetes diagnosis using neuro-fuzzy technique. Bulletin of Electrical Engineering and Informatics, 11(6), 3590-3597.
Azbeg, K., Boudhane, M., Ouchetto, O., & Jai Andaloussi, S. (2022). Diabetes emergency cases identification based on a statistical predictive model. Journal of Big Data, 9(1), 1-25.
Barakat, N., Bradley, A. P., & Barakat, M. N. H. (2010). Intelligible support vector machines for diagnosis of diabetes mellitus. IEEE transactions on information technology in biomedicine, 14(4), 1114-1120.
CDC (2021). What is Diabetes? Available at https:// www.cdc.gov/diabetes/basics/diabetes.html Accessed 2022-01-27.
Diabetes (2022). Available at https://www.who.int/news-room/fact-sheets/detail/diabetes Accessed 27 Jan 2022.
Ejim, E. C., Okafor, C. I., Emehel, A., Mbah, A. U., Onyia, U., Egwuonwu, T., ... & Onwubere, B. J. (2011). Prevalence of cardiovascular risk factors in the middle-aged and elderly population of a Nigerian rural community. Journal of tropical medicine, 2011.
Enang, O. E., Otu, A. A., Essien, O. E., Okpara, H., Fasanmade, O. A., Ohwovoriole, A. E., & Searle, J. (2014). Prevalence of dysglycemia in Calabar: a cross-sectional observational study among residents of Calabar, Nigeria. BMJ Open Diabetes Research and Care, 2(1), e000032.
Gezawa, I. D., Puepet, F. H., Mubi, B. M., Uloko, A. E., Bakki, B., Talle, M. A., & Haliru, I. (2015). Socio-demographic and anthropometric risk factors for type 2 diabetes in Maiduguri, North-Eastern Nigeria. Sahel Medical Journal, 18(5), 1.
Islam, M. M., Ferdousi, R., Rahman, S., & Bushra, H. Y. (2020). Likelihood prediction of diabetes at early stage using data mining techniques. In Computer vision and machine intelligence in medical image analysis (pp. 113-125). Springer, Singapore.
Katarya, R., & Jain, S. (2020, December). Comparison of different machine learning models for diabetes detection. In 2020 IEEE International Conference on Advances and Developments in Electrical and Electronics Engineering (ICADEE) (pp. 1-5). IEEE.
Laila, U. E., Mahboob, K., Khan, A. W., Khan, F., & Taekeun, W. (2022). An ensemble approach to predict early-stage diabetes risk using machine learning: An empirical study. Sensors, 22(14), 5247.
NCD Risk Factor Collaboration (NCD-RisC). (2017). Trends in obesity and diabetes across Africa from 1980 to 2014: an analysis of pooled population-based studies. International journal of epidemiology, 46(5), 1421-1432.
Oladapo, O. O., Salako, L., Sodiq, O., Shoyinka, K., Adedapo, K., & Falase, A. O. (2010). A prevalence of cardiometabolic risk factors among a rural Yoruba south-western Nigerian population: a population-based survey: cardiovascular topics. Cardiovascular journal of Africa, 21(1), 26-31.
Robinson, C. A., Agarwal, G., & Nerenberg, K. (2011). Validating the CANRISK prognostic model for assessing diabetes risk in Canada’s multi-ethnic population. Chronic Dis Inj Can, 32(1), 19-31.
Rubaiat, S. Y., Rahman, M. M., & Hasan, M. K. (2018, December). Important feature selection & accuracy comparisons of different machine learning models for early diabetes detection. In 2018 International Conference on Innovation in Engineering and Technology (ICIET) (pp. 1-6). IEEE.
Sabir, A., Ohwovoriole, A., Isezuo, S., Fasanmade, O., Abubakar, S., & Iwuala, S. (2013). Type 2 diabetes mellitus and its risk factors among the rural Fulanis of Northern Nigeria. Annals of African medicine, 12(4), 217.
Saeedi, P., Petersohn, I., Salpea, P., Malanda, B., Karuranga, S., Unwin, N., ... & IDF Diabetes Atlas Committee. (2019). Global and regional diabetes prevalence estimates for 2019 and projections for 2030 and 2045: Results from the International Diabetes Federation Diabetes Atlas. Diabetes research and clinical practice, 157, 107843.
Sarwar, A., Ali, M., Manhas, J., & Sharma, V. (2020). Diagnosis of diabetes type-II using hybrid machine learning based ensemble model. International Journal of Information Technology, 12, 419-428.
Shukla, A. K. (2020). Patient diabetes forecasting based on machine learning approach. In Soft Computing: Theories and Applications: Proceedings of SoCTA 2019 (pp. 1017-1027). Springer Singapore.
Swapna, G., Vinayakumar, R., & Soman, K. P. (2018). Diabetes detection using deep learning algorithms. ICT express, 4(4), 243-246.
Tinajero, M. G., & Malik, V. S. (2021). An update on the epidemiology of type 2 diabetes: a global perspective. Endocrinology and Metabolism Clinics, 50(3), 337-355.
Type 1 diabetes (2022) - symptoms and causes. https:// www.mayoclinic.org/diseases-conditions/type1diabetes/symptoms-causes/syc-203530119 Accessed 27 Jun 2022.
Type 2 diabetes (2022) - symptoms and causes. https:// www.mayoclinic.org/diseases-conditions/type2diabetes/symptoms-causes/syc-20351193 Accessed 27 Jun 2022.
Uloko, A. E., Musa, B. M., Ramalan, M. A., Gezawa, I. D., Puepet, F. H., Uloko, A. T., ... & Sada, K. B. (2018). Prevalence and risk factors for diabetes mellitus in Nigeria: a systematic review and meta-analysis. Diabetes Therapy, 9, 1307-1316.
Dada, E. G., Bassi, J. S., Chiroma, H., Adetunmbi, A. O., & Ajibuwa, O. E. (2019). Machine learning for email spam filtering: review, approaches and open research problems. Heliyon, 5(6).
Speiser, J. L., Miller, M. E., Tooze, J., & Ip, E. (2019). A comparison of random forest variable selection methods for classification prediction modeling. Expert systems with applications, 134, 93-101.
Taherkhani, A., Cosma, G., & McGinnity, T. M. (2020). AdaBoost-CNN: An adaptive boosting algorithm for convolutional neural networks to classify multi-class imbalanced datasets using transfer learning. Neurocomputing, 404, 351-366.
Chen, G., He, H., Zhao, L., Chen, K. B., Li, S., & Chen, C. Y. C. (2022). Adaptive boost approach for possible leads of triple-negative breast cancer. Chemometrics and Intelligent Laboratory Systems, 231, 104690.
Sharma, A., & Singh, B. (2020). AE-LGBM: Sequence-based novel approach to detect interacting protein pairs via ensemble of autoencoder and LightGBM. Computers in Biology and Medicine, 125, 103964.
Barrow, D. K., & Crone, S. F. (2016). A comparison of AdaBoost algorithms for time series forecast combination. International Journal of Forecasting, 32(4), 1103-1119.
Oyewola, D. O., Dada, E. G., Misra, S., & Damaševičius, R. (2021). Predicting COVID-19 cases in South Korea with all K-edited nearest neighbors noise filter and machine learning techniques. Information, 12(12), 528.
Oyewola, D. O., Dada, E. G. Exploring machine learning: a scientometrics approach using bibliometrix and VOSviewer. SN Applied Sciences, 2022, 4(5), 1-18. DOI: https://doi.org/10.1007/s42452-022-05027-7.
Dada, E. G., Yakubu, H. J., Oyewola, D. O. Artificial Neural Network Models for Rainfall Prediction. European Journal of Electrical Engineering and Computer Science, 2021, 5(2), 30-35.
Oyewola, D.O., Ibrahim, A., Kwanamu, J.A. Dada, E.G. A new auditory algorithm in stock market prediction on oil and gas sector in Nigerian stock exchange. Soft computing letters, 2021, 3, p.100013, https://doi.org/10.1016/j.socl.2021.100013.
Dada, E. G., Oyewola, D. O., Joseph, S. B., Duada, A. B. Ensemble Machine Learning Model for Software Defect Prediction. Advances in Machine Learning & Artificial Intelligence, 2021, 2(1), 11-21. https://doi.org/10.33140/AMLAI.02.01.03
Lingjun, H., Levine, R. A., Fan, J., Beemer, J., & Stronach, J. (2019). Random forest as a predictive analytics alternative to regression in institutional research. Practical Assessment, Research, and Evaluation, 23(1), 1.
Gieseke, F., & Igel, C. (2018, July). Training big random forests with little resources. In Proceedings of the 24th acm sigkdd international conference on knowledge discovery & data mining (pp. 1445-1454).
Xue, L., Liu, Y., Xiong, Y., Liu, Y., Cui, X., & Lei, G. (2021). A data-driven shale gas production forecasting method based on the multi-objective random forest regression. Journal of Petroleum Science and Engineering, 196, 107801.
Darlington, R. B., & Hayes, A. F. (2016). Regression analysis and linear models: Concepts, applications, and implementation. Guilford Publications.
Gomila, R. (2021). Logistic or linear? Estimating causal effects of experimental treatments on binary outcomes using regression analysis. Journal of Experimental Psychology: General, 150(4), 700.
Keele, L., Stevenson, R. T., & Elwert, F. (2020). The causal interpretation of estimated associations in regression models. Political Science Research and Methods, 8(1), 1-13.
Shafiee, S., Lied, L. M., Burud, I., Dieseth, J. A., Alsheikh, M., & Lillemo, M. (2021). Sequential forward selection and support vector regression in comparison to LASSO regression for spring wheat yield prediction based on UAV imagery. Computers and Electronics in Agriculture, 183, 106036.
Czajkowski, M., Jurczuk, K., & Kretowski, M. (2023). Steering the interpretability of decision trees using lasso regression-an evolutionary perspective. Information Sciences, 638, 118944.
Wang, S., Chen, Y., Cui, Z., Lin, L., & Zong, Y. (2024). Diabetes Risk Analysis Based on Machine Learning LASSO Regression Model. Journal of Theory and Practice of Engineering Science, 4(01), 58-64.
Rokem, A., & Kay, K. (2020). Fractional ridge regression: a fast, interpretable reparameterization of ridge regression. GigaScience, 9(12), giaa133.
la Tour, T. D., Eickenberg, M., Nunez-Elizalde, A. O., & Gallant, J. L. (2022). Feature-space selection with banded ridge regression. NeuroImage, 264, 119728.
Wang, L., Wang, Z., Qu, H., & Liu, S. (2018). Optimal forecast combination based on neural networks for time series forecasting. Applied soft computing, 66, 1-17.
Moon, J., Jung, S., Rew, J., Rho, S., & Hwang, E. (2020). Combination of short-term load forecasting models based on a stacking ensemble approach. Energy and Buildings, 216, 109921.
Mienye, I. D., & Sun, Y. (2022). A survey of ensemble learning: Concepts, algorithms, applications, and prospects. IEEE Access, 10, 99129-99149.
Wang, X., Hyndman, R. J., Li, F., & Kang, Y. (2023). Forecast combinations: an over 50-year review. International Journal of Forecasting, 39(4), 1518-1547.