Enhancing Smart Grid Efficiency through Machine Learning-Based Renewable Energy Optimization



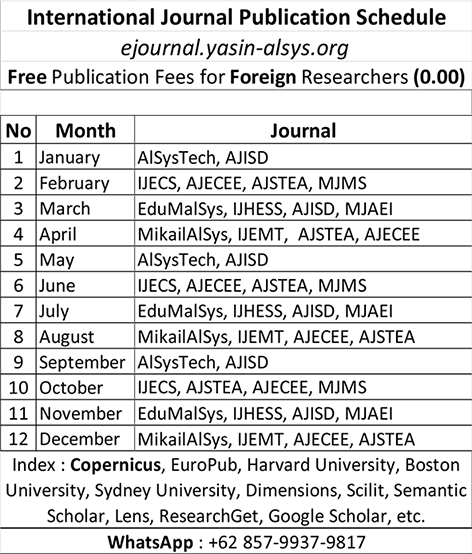
Please do not hesitate to contact us if you would like to obtain more information about the submission process or if you have further questions.
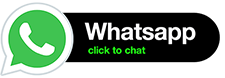
Abstract
Managing renewable energy in smart grids poses a significant challenge due to the inherent uncertainty and variability of energy sources like solar and wind power. To address this issue, we propose a novel approach that leverages the strengths of both Extreme Learning Machine (ELM) and Particle Swarm Optimization (PSO) algorithms. Our method utilizes ELM to model and predict renewable energy generation, enabling more accurate forecasting and planning. Meanwhile, PSO optimizes the parameters of the ELM algorithm, ensuring optimal performance and efficiency. We evaluated our approach using a dataset of solar energy production and compared its performance to existing optimization techniques. The results show that our ELM-PSO approach significantly improves the accuracy of renewable energy predictions and reduces energy costs in smart grids. The implications of our research are far-reaching, as our approach can be applied to various renewable energy systems, including wind turbines, solar panels, and hydroelectric power plants. By enhancing the efficiency and reliability of renewable energy utilization, we can create a more sustainable and resilient energy future.
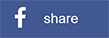
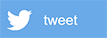

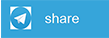
Citation Metrics:

Downloads
Authors retain copyright and grant the journal right of first publication with the work simultaneously licensed under a Creative Commons Attribution-NonCommercial-ShareAlike 4.0 International License that allows others to share the work with an acknowledgement of the work's authorship and initial publication in this journal.
References
Chen, X., Liu, J., & Li, Z. (2018). Machine learning for smart grid: A review. IEEE Transactions on Industrial Informatics, 14(4), 1734-1743.
Farhangi, H. (2010). The path of the smart grid. IEEE Power and Energy Magazine, 8(1), 18-28.
Intergovernmental Panel on Climate Change (IPCC) (2020). Climate Change 2020: Mitigation. Cambridge University Press.
Kiani, B., & Ghosh, P. (2019). Genetic algorithm for energy storage placement in a distribution network. Journal of Renewable and Sustainable Energy, 11(4), 043301.
Kim, J., Lee, Y., & Kim, B. (2019). Real-time monitoring and control of renewable energy sources using machine learning. Energies, 12(11), 2121.
Liu, Y., Wang, W., & Ghosh, P. (2019). Renewable energy management in smart grids: A review. Journal of Renewable and Sustainable Energy, 11(4), 043301.
Mao, M., Liu, J., & Wang, X. (2020). Machine learning for renewable energy management: A review. Energy Reports, 6, 123-135.
Santhi, G. B., Maheswari, D., Anitha, M., & Priyadharshini, R. I. (2023). Optimizing Renewable Energy Management in Smart Grids Using Machine Learning. In E3S Web of Conferences (Vol. 387, p. 02006). EDP Sciences.
Ullah, Z.; Wang, S.; Wu, G.; Xiao, M.; Lai, J.; Elkadeem, M.R. Energy Storage (2022), 52, 104814
Wang, Y., Chen, X., & Liu, J. (2019). Solar energy forecasting using machine learning algorithms. Energy Conversion and Management, 186, 112-121.
Yang, Y., Liu, J., & Li, Z. (2020). Deep learning algorithm for wind power generation prediction. IEEE Transactions on Smart Grid, 11(4), 2534-2543.
Zhang, Y., Liu, J., & Li, Z. (2020). Convolutional neural network for solar PV generation prediction. Energies, 13(11, 1338–1351.